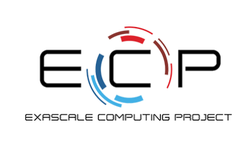
July 23, 2018
By: Michael Feldman
The US Exascale Computing Project (ECP) has set up its sixth co-design center, this one focused on machine learning (ML) technologies.
The new center, known as ExaLearn will target development of ML exascale software for science and engineering application projects and other work being performed under the ECP umbrella. Specifically, the aim is to produce a “scalable and sustainable ML software framework that allows application scientists and the applied mathematics and computer science communities to engage in co-design for learning.”
The effort will also include a collaboration with the Department of Energy’s (DOE) PathForward vendors to help develop this software for the various hardware platforms. These include processors and systems being developed by Cray, IBM, Intel, HPE, NVIDIA, and AMD.
Scalability is currently one of the biggest limitations for such software Even though the latest multi-teraflop GPUs can run machine learning codes rather efficiently, scaling applications beyond a handful of these devices remains a challenge. Getting this software to exascale, or even petascale is, for the most part, uncharted territory.
Although this machine learning software is being aimed at future exascale systems, it’s worth noting that there are two supercomputers at the DOE – Summit and Sierra – that can already execute such codes at this scale. Summit has the capacity to deliver more than 3 exaflops of deep learning performance, while Sierra can provide about 2 exaflops – in both cases derived from the custom Tensor Cores in their NVIDIA V100 GPUs. Summit has already run a comparative genomics code at 1.88 exaflops using this capability.
The practical outcome of the co-design work is to enable such applications to be developed for all exascale supercomputers, regardless of the underlying hardware, in the same manner as more traditional simulation and modeling codes.
The new center will include researchers and other experts from the eight core DOE national laboratories partnering with ECP, namely Brookhaven, Argonne, Lawrence Berkeley, Lawrence Livermore, Los Alamos, Oak Ridge, Pacific Northwest, and Sandia. Francis J. Alexander, Brookhaven’s Deputy Director of the Computational Science Initiative will be the principal investigator for the effort.
“Our multi-laboratory team is very excited to have the opportunity to tackle some of the most important challenges in machine learning at the exascale,” Alexander said. “There is, of course, already a considerable investment by the private sector in machine learning. However, there is still much more to be done in order to enable advances in very important scientific and national security work we do at the Department of Energy. I am very happy to lead this effort on behalf of our collaborative team.”